Until recently, the idea of first-degree price discrimination was just a theoretical concept resting in standard microeconomics textbooks, as the strategic implementation of such practices was often unfeasible. On the one hand, firms were unable to identify how much money each consumer would be willing to pay for the products they were selling. On the other hand, even if firms had such information, it used to be very costly (if not impossible) to successfully quote different prices to different consumers.
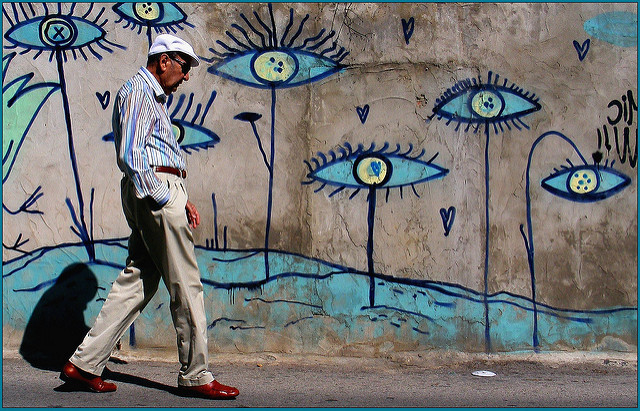
As already discussed on this blog, the situation is utterly different nowadays as we live the so-called Big Data Era. Indeed, firms may now potentially store tons of information on their consumers: what are the web pages they visit online, what is their purchase history for a significant number of products, consumers’ activity in social networks, the music/ videos consumers watch, consumers’ real-time location, and so on … Moreover, with all this information in their hands, firms may now rely on powerful algorithms to produce quite accurate predictions on consumers’ probability of buying a certain good and even on consumers’ willingness to pay for it. As mentioned by the data broker Omar Tawakol (boss of BlueKai, a company specialized in tracking consumers online and selling this information to companies) to The Economist (2014), nowadays firms’ success
“is 100% about having more information about the customer and being able to generate more commerce as a result of it.”
The availability of so much information on consumers’ characteristics together with the recent technological developments (allowing for personalized communication between firms and their customers) is also deeply changing firms’ pricing behaviour. In particular, this combination is allowing for the materialization of sophisticated price discrimination schemes, such as behaviour-based price discrimination personalized pricing, geo-pricing, geo-conquesting, …. Let us describe these different schemes.
Modern forms of price discrimination
Roughly speaking, price discrimination takes place when firms charge different prices to different consumers buying the same product. In the case of behaviour-based price discrimination, firms analyse consumers’ past purchase behaviour to infer their willingness to pay for the product and to price discriminate on the basis of this information. Another related concept is personalized pricing, which arises when firms charge a different price to each consumer in the market, depending on her specific features. For example, firms may send different discount coupons to consumers, depending on their purchase history (in that case, personalized pricing would actually be a form of behaviour based price discrimination). Other types of personalized pricing strategies include price discrimination schemes using information about consumers’ physical location, their online search behaviour, their social network activity, and so on. When firms track consumers’ location and price discriminate accordingly, personalized pricing takes the form of geo-pricing. For example, a firm may learn that a given consumer is approaching the rival’s store and send her a special discount so that she looses interest in visiting the rival (this particular form of geo-pricing is known as geo-conquesting).
Actually, the marketing jargon keeps growing as more and more firms take advantage of new digital technologies to successfully adopt price discrimination strategies. For instance, Adam Tenner (Forbes, 2014) alludes to an interesting study on personalized pricing in Spain covering 200 stores. This study found that
“if Internet visitors came from a discount site such as Nextag.com, they would at times receive prices as much as 23% lower than others.”
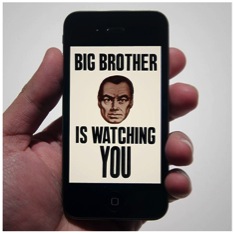
Along the same lines, the study also finds that prices of several stores (like e.g. Amazon, Staples, and the videogame store Steam) could fluctuate by as much as 166%, depending on consumers’ geographic position.
Dana Mattioli (Wall Street Journal, 2012) presents another interesting example of online strategies combining personalized product offers & pricing : Orbitz found out that, everything else the same, on average, Mac users spend as much as a 30% more on hotels than Windows users. The company has been using this information to show costlier online options to Mac users than the ones offered to Windows’ users visiting their website. When defining the search, the online platform also takes into consideration other aspects like hotel deals, the way at which consumers arrive at the website (e.g., arriving from a price comparison website is considered as a signal of higher sensitivity to prices than arriving from a quality ranking website), return visits and booking history as well as consumers’ location.
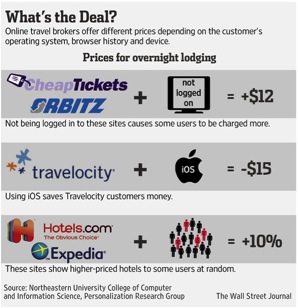
Airplane fares are also a good example of sophisticated pricing schemes involving price randomization. In the context of such strategies, firms randomly pick a price from a certain interval of prices, instead of always charging the same price, at each instant of time. This pricing behavior is often translated in unexpected momentary offers, as an attempt to prevent the consumers (and the rivals) from accurately predicting their prices, which is often the case with airplane online fares.
In 2014, the Department of Transportation in the USA has approved Resolution 787, which promotes the exchange of information in the air transportation marketplace. In addition, it“establishes certain goals associated with using the new technical standard, including
“establishes certain goals associated with using the new technical standard, including capability to provide personalized pricing offers to consumers who shop for air transportation.”
Resolution 787 illustrates firms’ concerns in creating the conditions to implement personalized pricing offers. However, while discussing this rule, several senators pointed out that consumers could be harmed by this process of exchanging information as airlines could use it to get extra-profits at the cost of consumers’ surplus:
“airlines could use that information to charge frequent business travelers higher prices. And there is also a concern that using ZIP codes or other demographic data might lead to discrimination that singles out groups based on their ethnicity, gender, or age for different prices.”
The previous examples show that, even when we only look at the effect of personalized pricing on consumers’ welfare (keeping aside important issues such as privacy concerns), it is not evident whether consumers are really benefiting from price discrimination or not. In particular, Christo Wilson (Northeastern University) explains that
“Here, there’s a transparency problem. The algorithms change regularly, so you don’t know if other people are getting the same results.”
While consumers generally appreciate receiving a special discount or reward, in a world of personalized pricing, consumers are only aware of their own price offer, which makes it difficult to assess the competitiveness of the personalized price offer they are receiving.
Do consumers benefit from personalized pricing?
In this context, Elizabeth Dwoskin (Wall Street Journal, 2014) raises the question: “Why You Can’t Trust You’re Getting the Best Deal Online?”, which leads us to another question: Do consumers benefit from personalized pricing?
The economics mainstream literature has stressed that in competitive environments with complete information, personalized pricing strategies could actually benefit consumers: firms’ attempts to poach each consumer in the market would end up dissipating their own profits away. This means that if firms were able to coordinate their pricing strategies they would actually prefer to commit to uniform pricing strategies rather than personalized pricing strategies (which would create a price war for each consumer in the market).
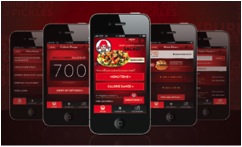
However, the analysis of firms’ price discrimination strategies in set-ups of incomplete information is not so straightforward (e.g. when consumers do not search enough or when they only know about the product upon receiving some ad on it). Esteves and Resende (2016) address this issue, studying firms’ optimal advertising and pricing strategies when firms serve distinct segments of consumers and they may target both ads and prices according to consumers’ preferences. They consider a very simple model in which two firms offer two differentiated products to two groups of consumers with distinct willingness to pay for each product.
In a context of informative advertising (meaning that consumers need to be exposed to advertising in order to be aware of the existence of the products), they find out that the interplay between targeted advertising and personalized pricing may end up relaxing price competition, namely when the consumers’ willingness to pay for both products is not too different (i.e. products are sufficiently close substitutes) and advertising costs are not too high.
In that case, firms may strategically reduce the intensity of ads targeted to the consumers with a large willingness to pay for their product (i.e., firms’ own strong market segment) with the objective of increasing the size of the potentially captive market of the firm selling the least preferred product in that segment. As a result, the last firm has incentives to relax price competition in order to get extra-profits from its captive consumers in the referred segment. Such consumers, despite preferring the other product, finally buy from their least preferred firm since they end up not being exposed to any ad from their favorite firm (who strategically chooses to advertise less in its strong market segment to sustain higher prices there).
In short, Esteves and Resende (2016) highlight two negative effects possibly (but not necessarily) arising as a consequence of the interplay between targeted advertising and price discrimination:
- Firms may successfully use this combination to sustain higher prices;
- There may be a considerable fraction of inefficient shopping (in the sense that consumers buy their least preferred product because they are uninformed about the existence of their favorite one).
In their stylized model, both outcomes are explained by firms’ strategic choice to reduce advertising to the strong market in detriment of advertising to the weak market.
These findings show that understanding the pros and cons of personalized pricing in digital markets is not an easy task. In particular, the interplay between consumers’ (lack of) information and firms’ pricing incentives may be quite intricate. In some cases, competitive price discrimination may actually be beneficial to consumers, who take advantage of firms’ “pricing wars” for each consumer. In other circumstances, that might not be the case and personalized discounts might just be a convincing label to mislead consumers on their assessment about the quality of the deal they are actually getting. |
The identification of the ingredients behind firms’ successful strategies to avoid consumer-poaching wars remains an open question, with important implications both on the side of firms (who need to design appropriate business models to make profits out of personalized pricing), on the side of consumers (who need to think strategically on the outcomes of their information revealing activities, like their online search behavior) and on the side of anti-trust authorities (who need to carefully scrutinize new sophisticated anti-competitive strategies made possible by the use of big data and digital technologies).
(Photo credits: gureu via Visual hunt CC BY-NC-ND / hpc-asia.com / WSJ /dmnews.com)