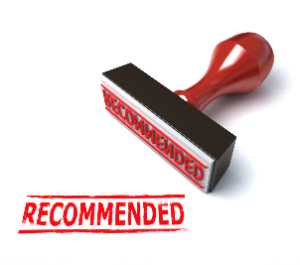
(Updated March 2015)
Earlier this month, Netflix (an American provider of on-demand Internet streaming media) offered some details about the working of its recommendation system. It is explained that recommendations are provided through various channels (“Top 10” lists, different genres appearing on the front page, ordering of movies) that are combined in a dynamic way. And apparently, the whole system is quite efficient: “The company says that its customers are so confident in the system at this point that 75 percent of all movies watched by members come from recommendations.”
Two weeks later, Splash.FM (a NewYork-based social music startup) announced its intention to push recommendations several steps further.
The site allows users follow friends and tastemakers in a Twitter-like fashion, share songs with their own network and “splash” the song recommendations of others to show their approval.
Users who “splash” a lot of songs and whose recommendations get reshared by others will over time accumulate a high “Splash Score,” which is something like a Klout score for your music curation skills.
It is of course too early to know how well this new recommendation system will perform, but it will certainly help users in finding more easily songs that match their tastes.
But which songs? It is indeed interesting to assess the effect that recommendation systems have on the sales distribution of existing products (and, eventually, their effect on the provision of variety). One may care, in particular, for niche products (i.e., products with a small market potential). As Chris Anderson has made it popular with his theory of the Long Tail, niche products are doing relatively better in the digital age so that the tail of the sales distribution becomes thicker (and longer). How does this long tail hypothesis withstand the spread of recommendation systems?
The question is far from simple. As explained in Belleflamme and Peitz (2010, p. 660):
While the long tail story refers to the diversity of aggregate sales, the discovery of better matches [made possible by recommendation systems] refers to diversity at the individual level. It might well be the case that people discover better matches through recommender systems but that they discover products which are already rather popular in the whole population. Hence, sales data in the presence of recommender systems may show more concentration at the aggregate level.
While this is an interesting insight, empirical analyses are needed to show whether recommendation systems indeed lead to more concentrated sales. One such, clever, empirical analysis is the one by Oesterreicher-Singer and Sundararajan (2010), who studied the influence of copurchase links on Amazon.com.
Here is a summary of their paper (see Case 23.3 in Belleflamme and Peitz, 2010, p. 661).
Oesterreicher-Singer and Sundararajan collected a large data set starting in 2005 of more than 250 000 books from more than 1400 categories sold on Amazon.com. They restrict their analysis to categories with more than 100 books. This leaves them with more than 200 categories. On all books, they obtain detailed daily information, including copurchase links, i.e., information on titles that other consumers bought together with the product in question (and which Amazon prominently communicates to consumers). These copurchase links exploit possible demand complementarities. Since these links arise from actual purchases and not statements by consumers, they can be seen as providing reliable information about what other consumers like. By reporting these links, Amazon essentially provides a personalized shelf for each consumer depending on what she was looking at last. This allows consumers to perform a directed search based on their starting point.
The question then is whether and how these copurchase links affect sales. In particular, the question is: which products make relative gains in such a recommendation network? Are these the products who already have mass appeal (because they are linked to other products) or rather niche products? To answer this question, one must measure the strength of the links that point to a particular product. For this it is important to count the number of links pointing to a product as well as the popularity of the products from which a link originates. Hence, a web page receives a high ranking if the web pages of many other products point to it or if highly ranked pages point to it. This is measured by a weighted page rank which is based on Google’s initial algorithm. The authors also construct the Gini-coefficient for each product category as a measure of demand diversity within a category. They regress this measure of demand diversity on the page rank (averaged within a category), together with a number of other variables. In their 30-day sample, they find that categories with a higher page rank are associated with a significantly lower Gini coefficient. This means that in a product category in which on average recommendations play an important role, niche products within this category do relatively better in terms of sales, whereas popular products perform relatively worse than in a product category where this is not the case. This is evidence in support of the theory of the long tail.
This post was originally written in 2012. Since then, recommendation systems have been improved and scholars in economics and in marketing have deepened our understanding of the effects that these systems can have on the sales distribution for various information goods (books, music, movies, …). The readers of this blog would undoubtedly appreciate any update on this issue. So, please provide them with a long tail of insightful comments 😉
(Past comments can be found here and here.)