How much would you be willing to pay in order to prevent your Internet provider from tracking and analysing your browsing history? According to AT&T, the Texas-based telecommunications provider, between $29 and $62 a month.
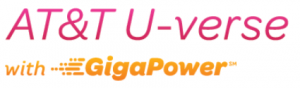
Back in December 2013, the company launched its new GigaPower service, which from summer 2014 onward is supposed to deliver one gigabit fast broadband connections. AT&T offers two different subscription plans that are based on a consumer’s preference for privacy. The standard service starts at $99 per month, but drops to $70 if the consumer agrees to let AT&T monitor his/her surfing habits. With video services, the price differential rises up to $62 a month (see, for example, here or here).
AT&T’s pricing model is build on two assumptions. First, consumers do not like having their surfing habits monitored. And second, they are willing to pay a monthly mark-up of more than 30% in order to prevent AT&T from tracking their browsing history.
This post is part of a three-piece series on the value of personal information in the digital economy. In this post, I will discuss whether consumers may actually benefit from sharing personal data with online service providers such as shopping websites, online news media, telecommunications providers and so on. The second part then introduces you to some basic notions of the Economics of Privacy. Finally, in the third part, I address the issue of how to price or more generally of how to value private information.
The benefits of sharing private information
In times of growing privacy concerns it appears an obvious thing to say that consumers do not like their surfing habits to be monitored. Nevertheless, I claim that this point is not as straightforward as it may appear at first glance – at least not from an economic point of view. In fact, as I will discuss in the following, consumers may actually benefit from sharing personal information, such as their browsing or purchasing history, with online service providers.
Behaviour-based price discrimination
In today’s digital economy, companies have a pretty good idea about who their consumers are. Indeed, the developments in information technologies allow companies to keep track of their customers’ search and purchasing behaviour and, based on this information, to develop detailed consumption profiles. Among other things, this availability of more and, in particular, better customer information makes the use of sophisticated price discrimination techniques possible. As such, companies may charge different prices to different consumers based on their past searches or purchases.
Contrary to the common perception, behaviour-based price discrimination does not necessarily make consumers worse off. Clearly, by definition, price discrimination implies that some consumers pay higher prices than others for one and the same good or service. However, it equally implies that some consumers pay a lower price. Further, the availability of more and more detailed information makes price discrimination more sophisticated. It is therefore more likely that consumers with a higher valuation for a product end up paying a higher price.
Also, as Acquisti and Varian (2005) point out, the absence of price discrimination does not necessarily imply lower prices.
Consumers are generally opposed to price discrimination because they think that if discrimination were not allowed they would end up with lower prices. However, as economists are happy to tell them, when discrimination is not allowed, all consumers may well end up facing higher prices. Policies that make customer tracking infeasible, such as enforcing the use of technologies for anonymous shopping, may well have the perverse effect of damaging consumer welfare for exactly the same reason: The profitable nondiscriminatory equilibrium ends up with monopoly pricing rather than competitive pricing.
Finally, behaviour-based price discrimination may yield positive externalities for consumers by increasing product variety. To see this, note that lower-valuation consumers may not be offered certain goods or services as their willingness to pay for the latter lies below the marginal cost of production. In this case, a premium paid by higher-valuation consumers may make it feasible to produce (or offer) those products to them. Higher-valuation consumers may thus subsidise lower-valuation consumers. To make things more concrete, let us have a look at Apple’s iPhone 5C and 5S. As some of you may know, the iPhone 5C is essentially a lower-price version of the iPhone 5S. In this context, one may argue that the higher price of the 5S version makes it possible to offer the lower-price 5C version to the segment of lower valuation consumers. Moreover, the availability of detailed consumer information is likely to have given Apple information on how to optimally differentiate the two versions regarding their features and prices.
Targeted advertising
The ability to monitor and analyse a consumer’s surfing habits not only allows a company to practice behaviour-based price discrimination, but also makes the use of targeted advertising techniques feasible. Paul Belleflamme explains the concept in this post on IPdigIT as follows.
When surfing the Internet, you must have noticed that the ads you are exposed to are closely connected to pages you visited or to items you bought (or considered buying) in the past. This is of course no coincidence: Internet advertising is increasingly targeted so as to raise the chances that the right person sees the right ad at the right time.
As argued in some of the comments to his post, there are several reasons for why consumers may be less opposed to this form of online advertising.
First, targeted advertisement may be more interesting. This is also the type of argument brought forward by AT&T.
When you select AT&T Internet Preferences, we can offer you our best pricing on U- verse with GigaPower because you let us use your individual Web browsing information, like the search terms you enter and the web pages you visit, to tailor ads and offers to your interests. You won’t necessarily receive more ads when you are online, but those you do see may be more suited to your interests.
Second, targeted advertisement may assist consumers when searching the web by providing them with relevant information. For instance, it may inform consumes about new, and most important, relevant offers that are related to their past purchases or search queries. Let me again refer to AT&T to provide you with some more concrete examples.
- If you search for concert tickets, you may receive offers and ads related to restaurants near the concert venue.
- After you browse hotels in Miami, you may be offered discounts for rental cars there.
- If you are exploring a new home appliance at one retailer, you may be presented with similar appliance options from other retailers.
As a corollary, targeted advertisement may equally facilitate the comparison of different online service providers such as online shopping sites. By this it may not only reduce a consumer’s search costs, but also increase competition between online service providers and hence put a downward pressure on prices.
Third, for a company, targeted advertising presents a more effective, or less wasteful, way to reach a large group of potential customers. In this context, Acquisti (2010) argues that the resulting increase in advertising revenues may be followed by a reduction in prices. In the same vein, higher advertising revenues may make the free provision of certain products feasible; just think of Facebook or Youtube. The information requirements of targeted advertising methods may consequently be seen as a necessary evil for the provision of innovative and high quality goods or services at reasonable prices.
Recommendation systems
Over the past decade recommendation systems have turned into an integral part of most e-commerce websites. Companies such as Netflix, the provider of on-demand Internet streaming media, analyse their customers’ past search or purchasing habits in order to be able to suggest items that a particular consumer is likely to enjoy. Bennett and Lanning (2007) provide some details on the working of Netflix’s recommendation system Cinematch and its information requirements.
The Cinematch recommendation system automatically analyzes the accumulated movie ratings weekly using a variant of Pearson’s correlation with all other movies to determine a list of “similar” movies that are predictive of enjoyment for the movie. Then, as the user provides ratings, an on-line, real-time portion of the system computes a multivariate regression based on these correlations to determine a unique, personalized prediction for each predictable movie based on those ratings.
What is in it for the consumer? A first and rather obvious point is that recommendation systems may help consumers to process the overwhelming amount of offers that are available online. As such, recommendation systems may facilitate search processes and make it easier to discover products that match one’s taste.
A not so obvious point is the relationship between recommendation systems and product variety. In Do recommendation systems make the tail longer or shorter Paul Belleflamme discusses the effect of recommendation systems on the sales distribution of existing products, in particular on the one of niche products. All in all, the effect of recommendation systems is far from obvious (I invite you to read the post and its comments). Nevertheless, there is empirical evidence that suggests that niche products do relatively better in the presence of recommendation systems (see, for example, Oestreicher-Singer and Sundararajan (2010)).
Single sign-on systems
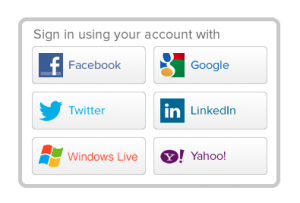
A recent study by Experian CreditExpert shows that the average person in the UK has 26 different online accounts and that moreover 17 percent sign up to at least six new accounts every month. With view on these figures it is intuitive that consumers may benefit from data sharing between different service providers if it reduces their costs of signing up across different online platforms. This is the idea behind so-called single sign-on systems, such as Facebook Connect or Google+ Sign In, that permit users to access multiple platforms with one set of login credentials. That is, the personal information a consumer shares with a particular online service provider may render the use of other online services more convenient or efficient due to data sharing between the different service providers.
The existence of such a secondary market for consumer data is further likely to entail benefits for online-based start-ups (and by this, indirectly for consumers, by increasing the variety of offers and the degree of competition between service providers). I will illustrate this point via the example of the multisided platform mind your own business school (MYBS) (see here for a case study on MYBS). MYBS assists future Master students in finding the perfect business school by enabling them to search, select and compare different Master programs offered by highly reputed European business schools. In general, anybody may browse MYBS, the use of certain website features, however, is conditional on the login via one’s Facebook or Linkedin account. The platform thus outsources any identity verification and relies on the controls of the two networking sites. For MYBS this not only implies lower administrative costs, but also that it may focus its time and efforts on the development or improvement of other website aspects.
Now that I described some of the potential benefits of sharing personal data I am interested in your opinion on this topic. What do you think? Do you benefit from letting online service providers accessing your personal data? Are there maybe other benefits than the ones I mentioned in the post? And do those benefits outweigh the costs of sharing personal information?